The Importance of Image Datasets for Object Detection
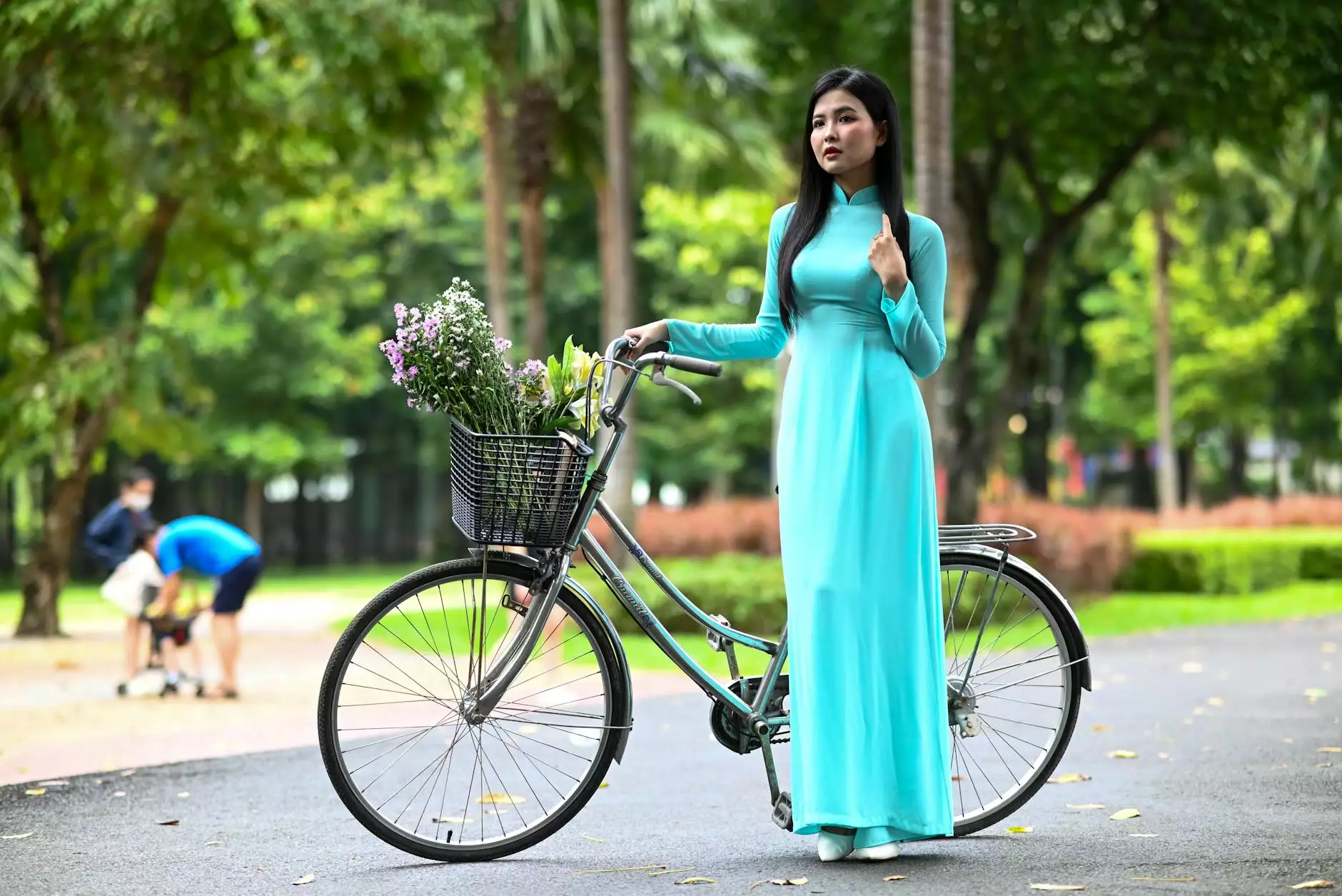
In the digital age, where the growth of artificial intelligence and machine learning continues to reshape industries, the use of an image dataset for object detection has become paramount. Whether it’s for enhancing automated quality control in manufacturing, real-time object recognition in video feeds, or improving augmented reality experiences, understanding how to efficiently curate and utilize datasets is critical for businesses aiming to stay competitive.
What is an Image Dataset for Object Detection?
An image dataset for object detection consists of a collection of images that are annotated with labels indicating the presence of specific objects within those images. This is essential for training algorithms that can accurately identify and localize objects in unseen images. The quality and comprehensiveness of these datasets directly affect the performance of machine learning models.
The Role of Data Annotation
Data annotation is the process of labeling data to provide context and meaning. In the realm of computer vision, this involves:
- Bounding Box Annotation: Drawing boxes around objects to indicate their location.
- Semantic Segmentation: Classifying each pixel in an image into categories.
- Instance Segmentation: Differentiating between individual objects of the same class.
- Keypoint Annotation: Marking specific points on objects, such as human joints in pose detection.
By annotating images meticulously, data scientists can build datasets that facilitate the training of robust object detection models capable of recognizing various objects in diverse scenarios.
Types of Image Datasets
When it comes to object detection, there are several types of datasets used, each serving distinct purposes:
1. Public Datasets
These are freely accessible datasets that contain a wide range of annotated images. Some popular public datasets include:
- COCO (Common Objects in Context): Contains over 330K images with annotations for object detection, segmentation, and captions.
- PASCAL VOC: Focuses on 20 different object classes with a well-defined benchmark for performance evaluation.
- ImageNet: Primarily used for image classification but has subsets available for detection tasks.
2. Custom Datasets
For businesses with specific needs, creating a custom dataset is often the best route. This involves:
- Collecting Data: Gathering images that represent the objects of interest.
- Annotating Data: Leveraging tools and platforms for efficient data labeling.
- Validating Data: Ensuring that the annotations are accurate and consistent.
The Process of Creating an Image Dataset
The creation of an image dataset for object detection follows a structured process. Here’s a detailed breakdown:
1. Define Objectives
Before diving into dataset creation, it is critical to define the objectives. What objects need to be detected? Understanding the scope will guide the data collection process.
2. Data Collection
Gather images from various sources, ensuring diversity in lighting, angle, and scenarios to enhance the model's robustness. Potential sources include:
- Stock Photo Websites
- User-Generated Content
- Company Databases
- Web Scraping (with proper permissions)
3. Data Annotation
Use a competent data annotation tool to streamline the process. At KeyLabs AI, we provide innovative solutions that allow for:
- Time-efficient annotations with advanced algorithms.
- High accuracy to minimize errors in labels.
- Team collaboration features that facilitate real-time updates and communication.
4. Data Validation
Once the dataset is annotated, it’s vital to validate the labels to maintain quality. This can include manual reviews or leveraging automated tools.
Why Quality Matters in Image Datasets
The impact of a well-prepared image dataset cannot be overstated. A quality dataset leads to:
- Improved Model Accuracy: The better the data, the more reliable the outputs.
- Faster Training Times: Efficient datasets reduce the computational resources required.
- Robustness in Real-World Applications: Training on diverse data ensures models perform well under various conditions.
Applications of Object Detection
Understanding the significance of an image dataset for object detection extends beyond data science; it translates into real-world applications:
1. Automotive Industry
In the development of self-driving cars, object detection is crucial for recognizing pedestrians, other vehicles, and road signs.
2. Retail and Security
Retailers use object detection for inventory management and improved customer experiences, while security systems utilize it for monitoring suspicious activity.
3. Healthcare
Medical imaging can be enhanced using object detection to identify anomalies in X-rays or MRIs, leading to timely interventions.
KeyLabs AI: Your Data Annotation Partner
At KeyLabs AI, we understand the complexities involved in creating an image dataset for object detection. Our data annotation platform offers:
- Scalability: Whether you have a handful of images or millions, our platform can accommodate your needs.
- Versatility: We support various annotation types to match your specific requirements.
- Expert Collaboration: Our team of experts ensures that your data is labeled accurately and expediently.
Conclusion
In conclusion, an image dataset for object detection is not just a collection of images; it is a cornerstone of technological advancement across various industries. By utilizing quality data annotation tools and leveraging platforms like KeyLabs AI, businesses can establish strong foundations for their machine learning initiatives. As the demand for high-performing object detection systems grows, ensuring you have the right datasets will set you apart from the competition.